Hi, my name is Chris, and I’m a machine learning guy at a tech company.
Everyone else: Hi Chris.
Better people than me have made the argument that technological complexity is an addiction with potentially disastrous long-term consequences. This week seems like a good time to talk about when things go wrong.
Not Giggling
note: this section has been updated with a statement from Giggle
“Accuracy” is a very context-dependent score. A baseball player who gets hits 35% of the time is an excellent hitter. At a quantitative hedge fund, being consistently right 51% (and levering up!) of the time is a license to print money. With extremely inbalanced classes, it becomes trivially easy to be “right”: the S&P will not drop 20% tomorrow, you will not click on the next ad you see, etc.
When we think about “accuracy” in machine learning, we often need to think about the business consequences of mispredictions, with either false positives or false negatives. Making a “bad” prediction for which photo to show at the top of an Instagram feed has relatively low risk, but using models in applications such as job applications have much larger human costs.
So there’s a “girls only social network” which claims to use “artificial intelligence” on selfies to gate entrance to only verified women. What could go wrong, indeed.
This is obviously a very nuanced subject and I want to specifically comment on the choice of using ML to determine gender only; I want to take the app’s creator at their word when they say they are inclusive to all who identify as female.
The app actually tells you to pose like passport photos, so I’d guess that they trained off a database of official photos and classified people as male/female. We often say that “a model is only as good as its training data,” so it’s already a bit of a red flag that they’re training on official ID photos and predicting on selfies, with much higher variation. If your pitch for the app is contingent on your model, you should probably think really hard about your business and the machine learning.
I think the app would have been basically fine if they did a similar verification as dating apps do, e.g. by linking social accounts in conjunction with the selfie, and taking more of a human approach to verification. Or even if they just said that there were humans in the loop. I’m sure that the more mature dating apps apply ML to the verification operations, but the ultimate user experience is very different when you believe a human is making the decison versus a “bone-scanning algorithm.” If they used the same algorithm, but enforced a 2-hour “processing” delay and dropped the phrenology, users probably wouldn’t have noticed!
UPDATE: statement from Giggle.
Creating a women’s only social networking platform should not be a controversial issue given the well documented levels of unsolicited abuse women receive in most areas of online activity.
Giggle’s mission is to create a platform where women can connect with women for a purpose, free from misogynistic abuse.
We believe that all women are entitled to a space where they can communicate, network, meet new people, and discuss issues without the threat of piled on abuse.
We have designed the platform to create micro-groups, up to 6 people, where everyone joins with the consent of the others. We have categories ranging from “gender identity support” to “political activism” to “writing gigs”.
In order to carry out our primary mission of creating a female only space, we have chosen facial recognition as the first gatekeeper. At giggle HQ, real people observe the gatekeeping process, and if and when errors occur, they correct them.
Facial recognition software detects gender assigned at birth, with a high degree of accuracy although nothing is infallible. That is why we monitor the process with real people on the screens and have worked with the LGBTQ+ community to ensure that gender identity is an equal part of giggle despite any technological shortcomings. The facial recognition is detecting men, not judging women, to ensure that men can not get on the girls only app.
A user is required to supply their mobile phone number and a selfie for gate keeping purposes. They may use a pseudonym, and are not required to verify date of birth or age, provide their address or any other information that can be used to identify them. The only data that we collect and use is to ensure the functionality of the app.
The threat of abuse online is far too big for any one person remedy. This is why we have created a refuge from it.
The true controversy is the misogynistic, transphobic, racist and bigoted abuse women experience online and in the real world every day.
My followups and responses (SG is the founder of giggle):
CH: You say, "No cats have gotten on to giggle. No men have gotten on to giggle.”
SG: As stated in the statement, the technology is backed up by human beings. The software has a “liveness” test - so no users trying to get using a photograph of a girl can get on. Thousands have tried using a photograph of me. It has not worked.
CH: You additionally say, "Facial recognition software detects gender assigned at birth, with a high degree of accuracy although nothing is infallible." Would you like to comment further on how you are measuring that accuracy, and how you can make definitive statements that there are no "men" on giggle, when the technology is fallible?
SG: The verification software is looking for men, to ensure that no man is getting on to giggle. It actually isn’t looking for females at all - hence why we stated that there could be an element of difficulty for trans girls. The connection there should be obvious, rather than thinking we’re judging anyone to see how “feminine” they are, which is not the case. Again, as stated in the statement, the technology is backed up by human beings, so if a mistake has been made (on an extremely rare instance, at this point), it can be rectified ASAP. There are no men (or cats) on giggle.
The verification software is AI that has learned the difference between a male and female appearance based on millions of photographs.
Caucusin’ Around
Obviously I am late to the game on the Iowa caucus hot takes. I trust that other people have providing sufficient takes?
“We should never ever use technology in an election” was a pretty popular take this week. I think that that’s not fair, given that previous iterations of the caucus also had an app, developed by Microsoft, instead and things were “fine.” I mostly think the reason the caucus appeared to go poorly was because the tech was just developed incompetently by Shadow, rather than actual malice (which I won’t rule out). But more on that later.
The hottest take I’ve seen has been from the Iowa Democratic Party’s lawyers:
“The I.D.P.’s role is to facilitate the caucus and tabulate the results,” Ms. McCormally continued. “Any judgment of math miscalculations would insert personal opinion into the process by individuals not at the caucus and could change the agreed upon results. That action would be interfering with the caucus’ expression of their preferences. There are various reasons that the worksheets have errors and may appear to not be accurate, however changing the math would change the information agreed upon and certified by the caucusgoers.”
Sure, ok.
My time in tech has taught me some useful frameworks to think about the caucus, however.
You could think about the electoral process as a product, where your users are the voting populace, and their main Job To Be Done is have their electoral preferences be heard. In that case, you’d want to reduce voter friction wherever possible. You probably wouldn’t design a caucus, which requires a lot of voter time, but even within the caucus framework, you’d think of the precinct captains as being customers too and design for their job of “calculating and reporting results accurately.” I tweeted that the app should have been a Google Form and I stand by that: forms are familiar to the end users, are as secure (file reports with a verified Gmail login), and would reduce the amount of necessary math.
But if you think about the electoral process as being designed for the party leaders, whose function is to preserve their current positions and power, then the systems make sense. The rules are complex and arbitrary, and as we’ve seen, are basically up to the party’s interpretation. The more work that appears to be done, the more valuable the state party seems, to the national party and to the politicians, who now have incentives to send money to those states’ parties.
The precincts used to only report final state delegate counts, which is the equivalent of “not showing your work” on a math exam. Rigging an election is significantly more difficult without information asymmetry, and the increased information available this time means that people were able to properly audit the results.

There’s no reason to believe that previous caucuses, which went comparatively “smoothly,” were correctly calculated either. I have gotten significantly worse at math over the last four years, since leaving college, but I refuse to believe that that’s true for everyone else. Did the Microsoft-built app check for mathematical mistakes, or did it also just submit whatever was inputted? We don’t have the information, and the IDP won’t tell us.
Technology is not neutral, it is built for a purpose and an end-user in mind. Was the Microsoft app really better than the Shadow app? Sure, it ran more smoothly and tabulated numbers more quickly. But if that’s all the app did, it also whitewashed the mistakes of its end users and didn’t provide faithfully represent the population. In a way, I’m thankful that the shitty app got people to dig deeper and figure out the actual source of the error - humans.
In a simpler and transparent election, there would be significantly less need for interpretation and less need for the state party apparatus. The information asymmetry and complexity allows the IDP and the Democratic Party to maintain control over their processes. In the corporate world, this control tends to manifest itself as barriers to entry and higher margins, but the corporate world offers many examples of companies successfully “disintermediating” others and disrupting their industries. Maybe that’s what we need in politics.
Elections and gender identity are both places where getting the outcome wrong has significant costs and where our decision-making process deserves lots of scrutiny. Ultimately, we’re human and make mistakes; how can we expect tools built for humans to “fix” all of that behavior? I think the answer is in recognizing our flaws (and the potential for bad actors), and designing systems which then serve the right set of constituents: for example, voters, not parties.
BONG!
Like most other observers, I expected 1917 to win Best Picture over Parasite, a fantastic, genre-defying movie which presents the most nuanced depiction of class struggle that I’ve ever seen on film. Watching the film and its director sweep all four awards they were nominated for, including Best Director and Best Picture, was a pretty good moment. Let me extemporize a bit about why the win felt so nicely juxtaposed.
World War One was a bad war. There was no good or bad side; there were victors and losers, but there were no lessons learned or moral high ground to stand on. So movies about WWI always puzzle me — why should I root for the utterly immoral British empire versus the just as immoral German empire? Wonder Woman was another recent film before 1917 to present this same narrative, of the Allies in WWI somehow being morally superior to their enemies and worthy of divine intervention.
Comparatively, Parasite has no villains, has an unfamiliar setting, and defies the easy packaging seemingly necessary to win. Korea, that unfamiliar setting, is a country with a very long history of colonialism, most recently by the Japanese and Americans. Much of modern Korean cuisine, for example, has been born out of that combination: “soldier soup” is made from ramen (Japanese) and spam (American) and “corn cheese” is made from the American cheese, also left over from the US Army. Fundamentally, these are dishes of poverty and necessity, as many dishes are, but belie Korea’s long culinary heritage. Korea is culturally having a moment, with BTS quite possibly being more popular on Twitter than Trump, but much of the media narrative has been focused on the “crazy rich” Koreans rather than the working class, for whom future prospects are extremely poor and who face the second highest rate of suicide in the OECD. Everyone talks about film not telling stories about the right people, so it’s nice to see the right things win for once.
Congratulations to the crew and cast! Geonbae!
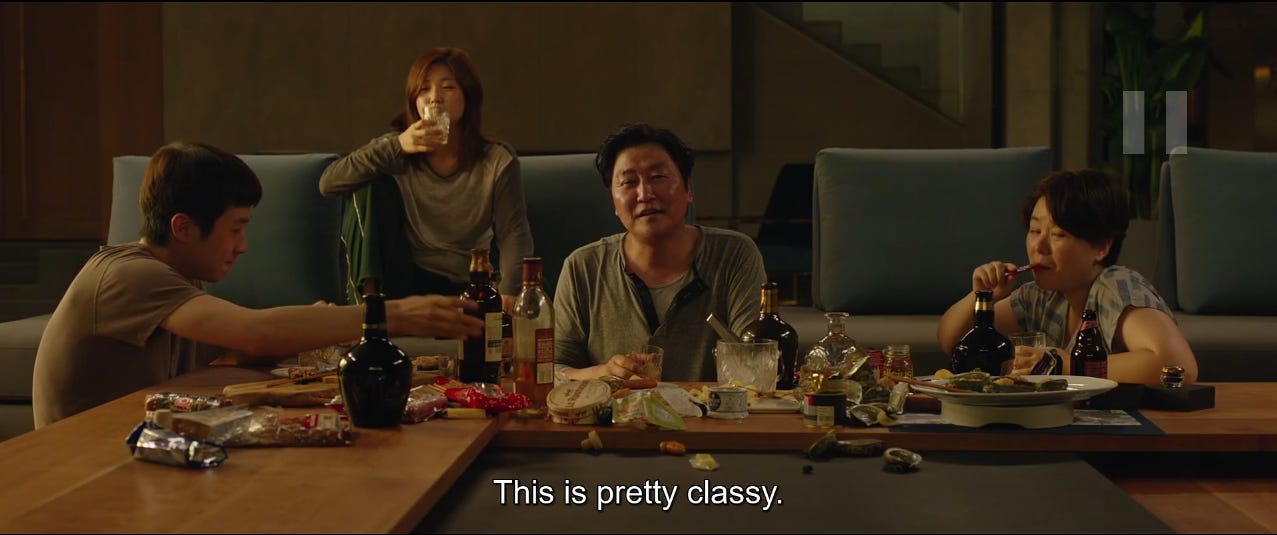