If the big AI labs are right, we will get to a point soon where LLM's can create human-level or better work on most white collar tasks. Separately, the robotics space is heating up, with all parts of the humanoid robot stack getting fleshed out. Let's assume these bets work out.
Will AI augment or automate us? I posit that the pivotal characteristic is if it matters *who* presents the work product. If the identical work, presented by two different people, results in a different result, then AI must augment the human, not automate. In other words, the only durable and valuable human work is subjective in nature.
where are we now
Let's first briefly market map, then think about some ideas for differentiation.
It's very much consensus at this point that lots of lower class work will be automated. Marketplace apps have already created the notion that every provider is commoditized and taken over the customer relationship. Think TaskRabbit, Doordash for restaurants, Uber for cabs, Waymo for Uber, so on. I wrote more about this 5 years ago and think that the piece held up very well.
But what about white collar work?
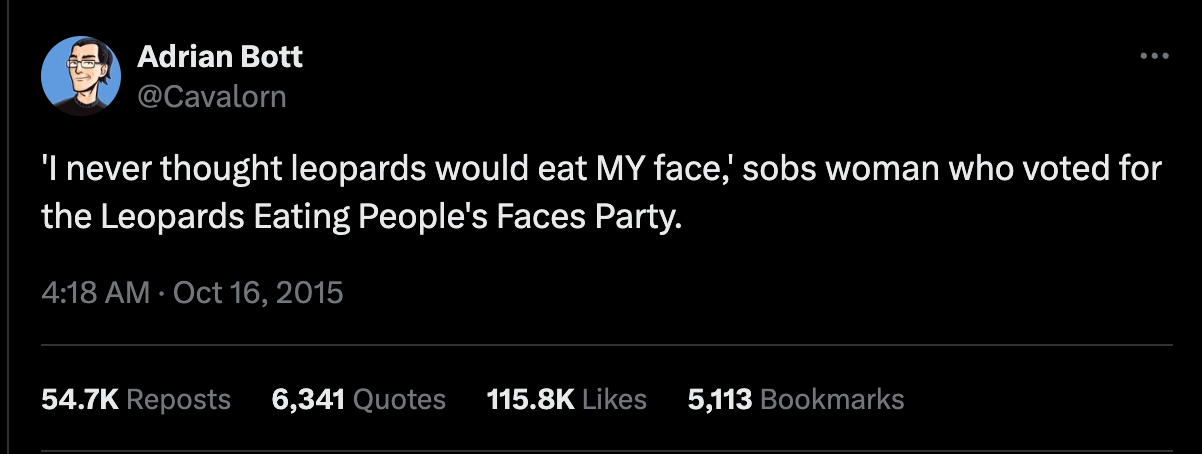
The same heuristic applies: does it matter who presents the output?
Clay is a good example of the dichotomy here. They offer a product that aggregates data vendors and uses LLM's to synthesize a cold outreach sales email, or basically what an entry-level sales development representative (SDR) does. This process augments a more senior account executive (AE), who hits send on the outreach, and it automates the more junior SDR work. The AE’s appearance, network, and delivery matters a lot to the actual sale, but the SDR’s sourcing and data research is internal and commoditized. It feels pretty bad to have an AI do the actual sales pitch.
Some professions, for example paralegals, are prohibited from presenting work to clients. They do all the research and drafting but all of their work product must be presented internally and only a licensed attorney can present it to a client. This kind of work seems to be in a very tough spot: surely there are good and bad paralegals, but good luck making that distinction.
Most junior software engineering tasks are also quite simple with little room for judgement - bugfixes, patches, small features, so on. The skill is explaining exactly what the business requirement for a task is, what a PM or tech lead might do.
Does it matter which person pitches a new product feature to leadership? I am biased, as a former data scientist and product manager, but I'd think so. A/B tests are totally gamable and very far from a science. I'll give one example: a major app you likely use redesigned their entire UX and saw very negative engagement results from a small experiment. The head of design's job depended on shipping the redesign, so they convinced their data scientists to manually exclude every user that churned; they argued that the big drops were because of bugs that would be fixed in the real launch. If you exclude everyone that stops using the app from your treatment, of course the results will look great! Might the job of setting up and running an experiment be automated? Yes. Will the job of using data to lie be automated? No.
what’s next
There's 3 main strategies I can think of for not being obsoleted by these trends:
Do less legible jobs. What does a VC or PM actually ... do? What differentiates a great salesperson from an average one? If you can't tell what they're supposed to do, or it changes all the time, that's harder to automate. Shape rotators cope and seethe.
Get better at storytelling. This could be working on presentation skills, or on a more base level, "looksmaxxing" - becoming more attractive to stand out.
Work in a regulated industry or for the government. The number one goal of government and politicians is to stay in power, and strong governments generally act very quickly to make sure citizens have jobs (unemployed people have more time to protest and riot). I expect the government to expand in size or tie "human-made" requirements to government contracts or state funding (fiscal federalism).
Aswath Damodaran (the valuation prof) came to similar conclusions recently: tasks with objective outcomes are easiest to automate and those with subjective outcomes are hardest, and that regulation is a source of protection.
There's a few historical examples I want to study. One is the history of retail and marketing - how do CPG companies differentiate themselves? Another is the Korean beauty industry - how do individuals in a low-growth economy stand out? Would love book recommendations!